13 ways technology enables people-first change orchestration
- Leslie Ottavi
- May 2, 2023
- 5 min read
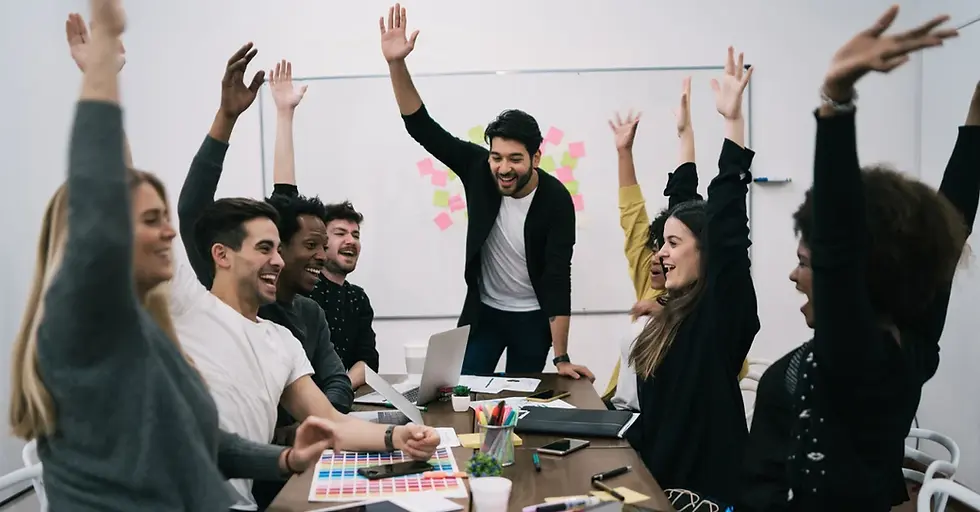
Incorporating data analytics, and automation to the change model has been among the most disruptive evolutions to the practice of enterprise transformation that I’ve seen in my 15-year career. Change leaders systematically tell us, “This is just what I have needed all these years. This is a game-changer!”
If you’re reading this as a transformation leader, change manager, or portfolio manager, you know that typically, business initiatives are initiated by enterprise leadership. Enterprise leaders set the vision and goals and expect organization and portfolio managers to align their own goals and projects to the enterprise objectives.
Teams up and down the enterprise hierarchy kick off initiatives to drive solutions to achieve the business outcomes that are expected.
As a result, a lot of people need to change the way they work, all while they are busy running the business. The trouble is that the people who need to change are traditionally considered during the last mile of the transformation initiative.
While the folly of this is obvious since the initiative’s very success depends on the willingness and ability of stakeholders to change, it hasn’t been easy to achieve the necessary paradigm shift to do things differently, especially in a medium- or enterprise-sized company.
Until now. Artificial intelligence, data analytics, and automation have come a long way in recent years, and offer change leaders phenomenal tools and insights not only for facilitating and accelerating adoption, but also for tangibly demonstrating the value of change management and empowering leadership to consider initiatives from the stakeholder perspective.
Siloed approaches cloud the stakeholder view
One of the biggest challenges in enterprise change management is understanding change breadth, complexity, and risk across multiple portfolios. Organization A understands what it needs to do, whom it needs to coordinate with and maybe even how much their stakeholders will be impacted.
Organization B is implementing initiatives of its own in parallel, some of which may impact the same stakeholder groups as Organization A.
These different initiatives keep layering on top of each other. Enterprise-sized companies often have dozens of initiatives in flight at any given time. The more organizations are involved, the more saturated the change becomes.
Because each organization tends to manage its initiatives in a silo, they don’t necessarily have visibility into what the other organizations are doing, except perhaps for some specific programs.
Since each organization uses its own change management approaches, stakeholders often have inconsistent experiences with the different initiatives affecting them, which leads to the feeling of activity overload and change fatigue.
As a result of this siloed approach, a holistic view of the cumulative change breadth, complexity and risk across the enterprise is unavailable. At the same time, change leadership is supposed to be making decisions about what should be done, and when.
Traditional reporting tools are not scalable
Typically, change managers use tools like templates, assessments, and spreadsheets to gather information about their initiatives. They then manually transform and load the data into visualization tools like Power BI or Tableau to graphically represent what is happening.
When we consider that nearly half of change managers report* managing at least three change initiatives at a time (and more than 10% manage 10 or more!), all that manual extraction, transformation and loading becomes unfeasible.
Many organizations hire contract workers just to keep up with all the data ETL needed to report on their change initiatives, and even then, the information on each report is limited to a specific project.
Enterprise leaders, then, rely on disparate information from distinct portfolios presented in varying formats to try to piece everything together to understand the big picture of what is changing, who is impacted, and what is being done to get teams ready across the enterprise.
With this much complexity and inconsistency in the implementation and reporting of change initiatives, it is no wonder that stakeholders tend to get lost in the shuffle and considered late in the project life cycle!
Automation and data analytics enable stakeholder-specific views
Recent developments in data analytics and automation enable change leaders to get actionable insights from data about their projects at every vantage point, from the individual stakeholder level all the way up to the enterprise level.
Once everyone impacted by your project – whether it be at the -C-suite level, the organization level, the portfolio level, the program level, or the individual end-user level – can clearly see how, when and why your project will affect them specifically, the dialogue changes.
It’s no secret that people don’t engage with things they don’t perceive as relevant to them. But when it’s obvious how a project will directly affect them and their teams, engagement comes naturally.
AI, data analytics and automation also enable you to scale and include information about all of the other initiatives across the enterprise into your model, so that reporting becomes holistic and change plans become coordinated.
When your reports are telling you that of the 10 initiatives in flight, six of them affect the marketing department, conversations can happen across programs and portfolios to coordinate the deployment of the action plans so that end users are not overwhelmed.
13 benefits of applying data analytics and automation to the process of change management
Once you can capture and manipulate data about all the change initiatives across the enterprise from multiple perspectives, you can customize your reports to each stakeholder to stimulate engagement, promote adoption, and create a change-embracing culture across the enterprise. As a result:
Your reports focus specifically on information and insights relevant to a specific stakeholder (end user, portfolio, organization, leadership, etc.).
Reporting is holistic and includes information about initiatives across portfolios.
Change plans can be coordinated, since information about how the initiatives across the enterprise cumulatively affect each stakeholder.
Decisions about what, how and when to deploy are data-driven.
Leadership has a holistic understanding of the budget and resources needed to drive change.
Reports are generated automatically, saving valuable time and resources.
Rather than spending time generating reports, change leaders’ time can be spent on people-first tasks like engaging with leadership and their stakeholders.
Holistic views about what is changing, who is impacted and what is being done to get teams ready enable more meaningful dialogue between otherwise siloed portfolios.
A culture of change is promoted; people are more ready and willing to adopt change.
Change initiatives experience better and faster adoption.
Change metrics and ROI are clearly understood.
The change model is scalable and can incorporate data from an unlimited number of projects.
AI takes insights from past initiatives and intelligently suggests actions for current initiatives, which future-proofs your change model by enabling it to evolve as the enterprise changes and grows.
If you have incorporated automation and data analytics into your change model, share in the comments what other outcomes you have experienced beyond the ones I’ve listed here!
留言